Trust and Security: Difference between revisions
Line 21: | Line 21: | ||
Safety traditionally is closely associated with Verification and Validation, which has its own, dedicated section in Ignite AIoT. The same holds true for robustness (see Reliability and Resilience). Since security is such a key enabler, it will have its own, dedicated discussion here, followed by a summary of AIoT Trust Policy Management. Before delving into this, we first need to understand the AI and IoT-specific challenges from a security point of view. | Safety traditionally is closely associated with Verification and Validation, which has its own, dedicated section in Ignite AIoT. The same holds true for robustness (see Reliability and Resilience). Since security is such a key enabler, it will have its own, dedicated discussion here, followed by a summary of AIoT Trust Policy Management. Before delving into this, we first need to understand the AI and IoT-specific challenges from a security point of view. | ||
__TOC__ | |||
== AI-related trust and security challenges == | == AI-related trust and security challenges == |
Revision as of 19:11, 14 September 2020
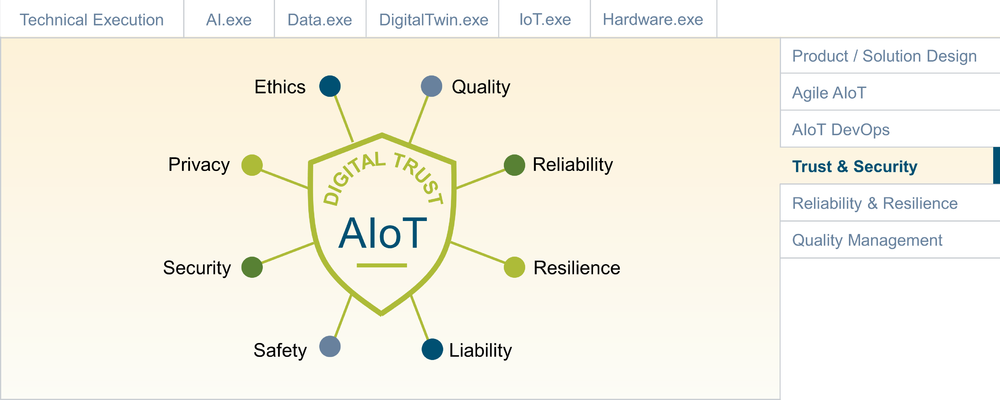
Ignite AIoT: Trust & Security
Digital Trust – or trust in digital solutions – is a complex topic. When do users deem a digital product actually trustworthy? What if a physical product component is added, as in smart, connected products? While security certainly is a key anbler of Digital Trust, there are many other aspects which are important, including ethical considerations, data privacy, quality and robustness (including reliability and resilience). Since AIoT-enabled products can have a direct, physical impact on the well-being of people, safety also plays an important role.
Safety traditionally is closely associated with Verification and Validation, which has its own, dedicated section in Ignite AIoT. The same holds true for robustness (see Reliability and Resilience). Since security is such a key enabler, it will have its own, dedicated discussion here, followed by a summary of AIoT Trust Policy Management. Before delving into this, we first need to understand the AI and IoT-specific challenges from a security point of view.
As excited as many business managers are about the potential applications of AI, as sceptical are many users and citizens. A key challenge with AI is that it is per-se not explainable: There are no more explicitly coded algorithms, but rather „black box“ models which are trainined and fine-tuned over time with data from the outside, with no chance of tracing and „debugging“ them the traditional way at runtime. While Explainable AI is trying exactly this challenge, there are no satisfactory solutions available yet.
One key challenge with AI is bias: While the AI model might be statistically correct, it is being fed training data which includes a bias, which will result in – usually unwanted – behaviour. For example, an AI-based HR solution for the evaluation of job applicants which is trained on biased data will result in biased recommendations.
While bias is often introduced unintentionally, there are also many potential ways to intentionally attack and AI-based system. A recent report from the Belfer Center describes two main classes of AI attacks: Input Attacks and Poisioning Attacks.
Input attacks: These kind of attacks are possible because an AI model never covers 100% of all possible inputs. Instead, statistical assumption are made and mathematical functions are developed to allow creation of an abstract model of the real world, derived from the training data. So-called adverserial attacks try to exploit this by manipulating input data in a way that confuses the AI model. For example, a small sticker added to a stop sign can confuse an automous vehicle and make it think that it is acually seeing a green light.
Posioning attacks: This type of attack aims at corrupting the model itself, typically during the training process. For example, malicious training data could be inserted to install some kind of back-door in the model. This could, for example, be used to bypass a building security system or confuse a military drone.